RoScenes
A Large-scale Multi-view 3D Dataset for Roadside Perception
1Alibaba Cloud,
2Sichuan Digital Transportation Technology Co., Ltd,
3Independent Researcher,
4Tongji University
*Equal contribution, †Project lead, ‡Corresponding authors
We introduce RoScenes, the largest multi-view roadside perception dataset, which aims to shed light on the development of vision-centric Bird's Eye View (BEV) approaches for more challenging traffic scenes. The highlights of RoScenes include significantly large perception area, full scene coverage and crowded traffic. More specifically, our dataset achieves surprising 21.13M 3D annotations within 64,000 m2. To relieve the expensive costs of roadside 3D labeling, we present a novel BEV-to-3D joint annotation pipeline to efficiently collect such a large volume of data. After that, we organize a comprehensive study for current BEV methods on RoScenes in terms of effectiveness and efficiency. Tested methods suffer from the vast perception area and variation of sensor layout across scenes, resulting in performance levels falling below expectations. To this end, we propose RoBEV that incorporates feature-guided position embedding for effective 2D-3D feature assignment. With its help, our method outperforms state-of-the-art by a large margin without extra computational overhead on validation set.
Sichuan Expressway Construction & Development Group Co., Ltd.
Western Sichuan Expressway Co., Ltd.
Sichuan Intelligent Expressway Technology Co., Ltd.
for their invaluable assistance with data acquisition.
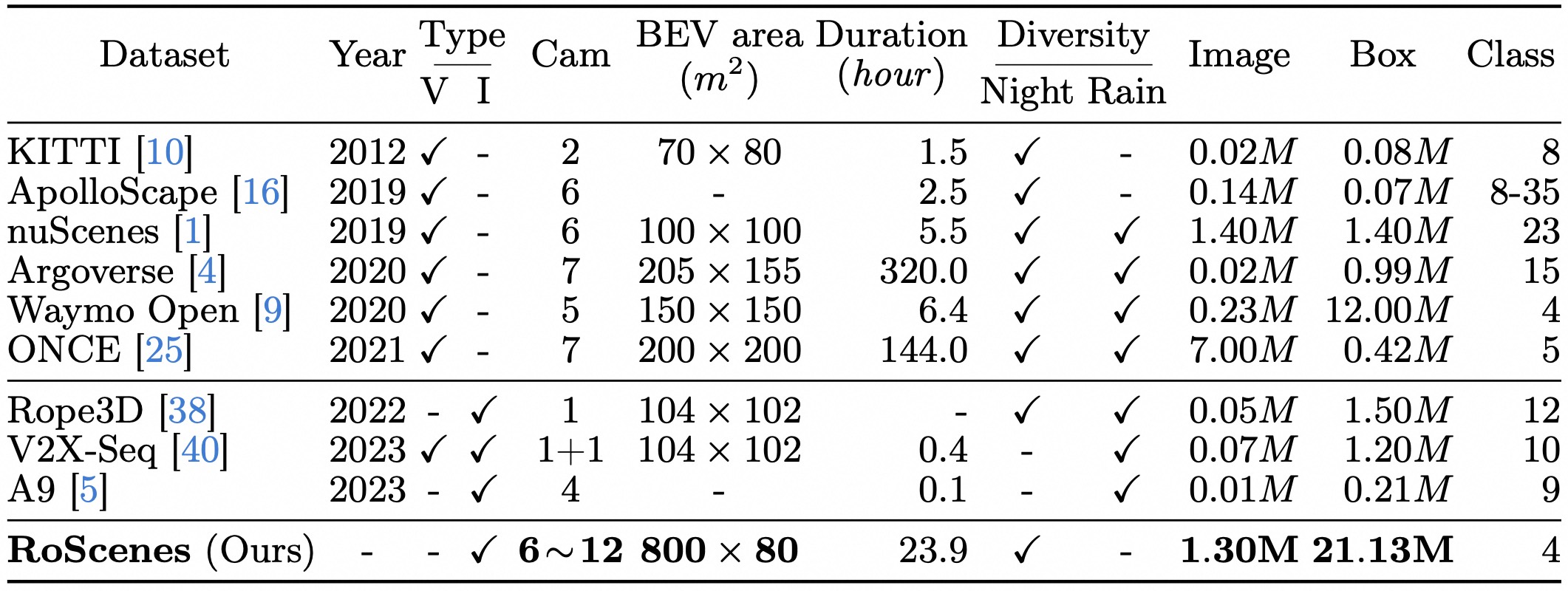
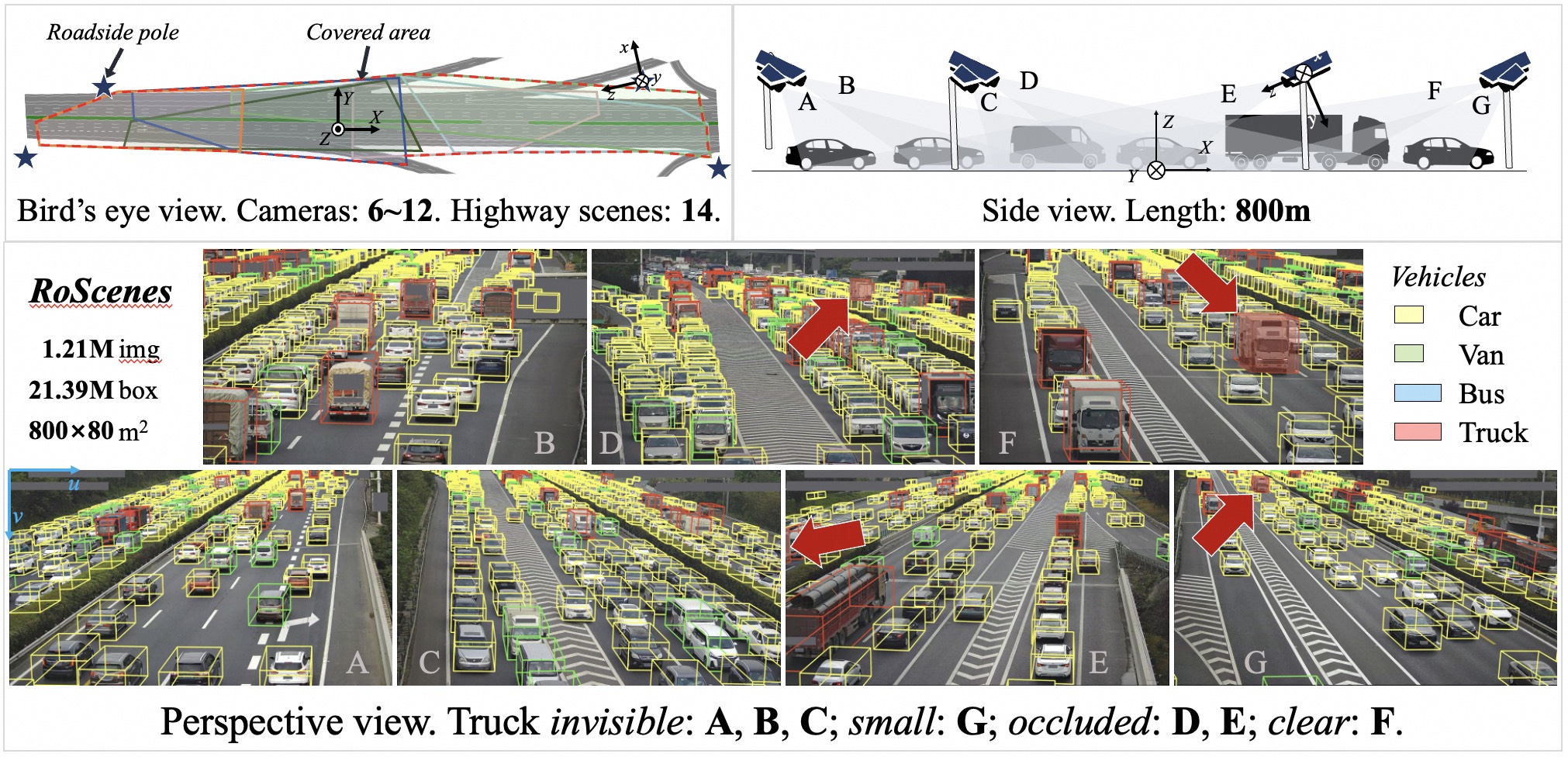
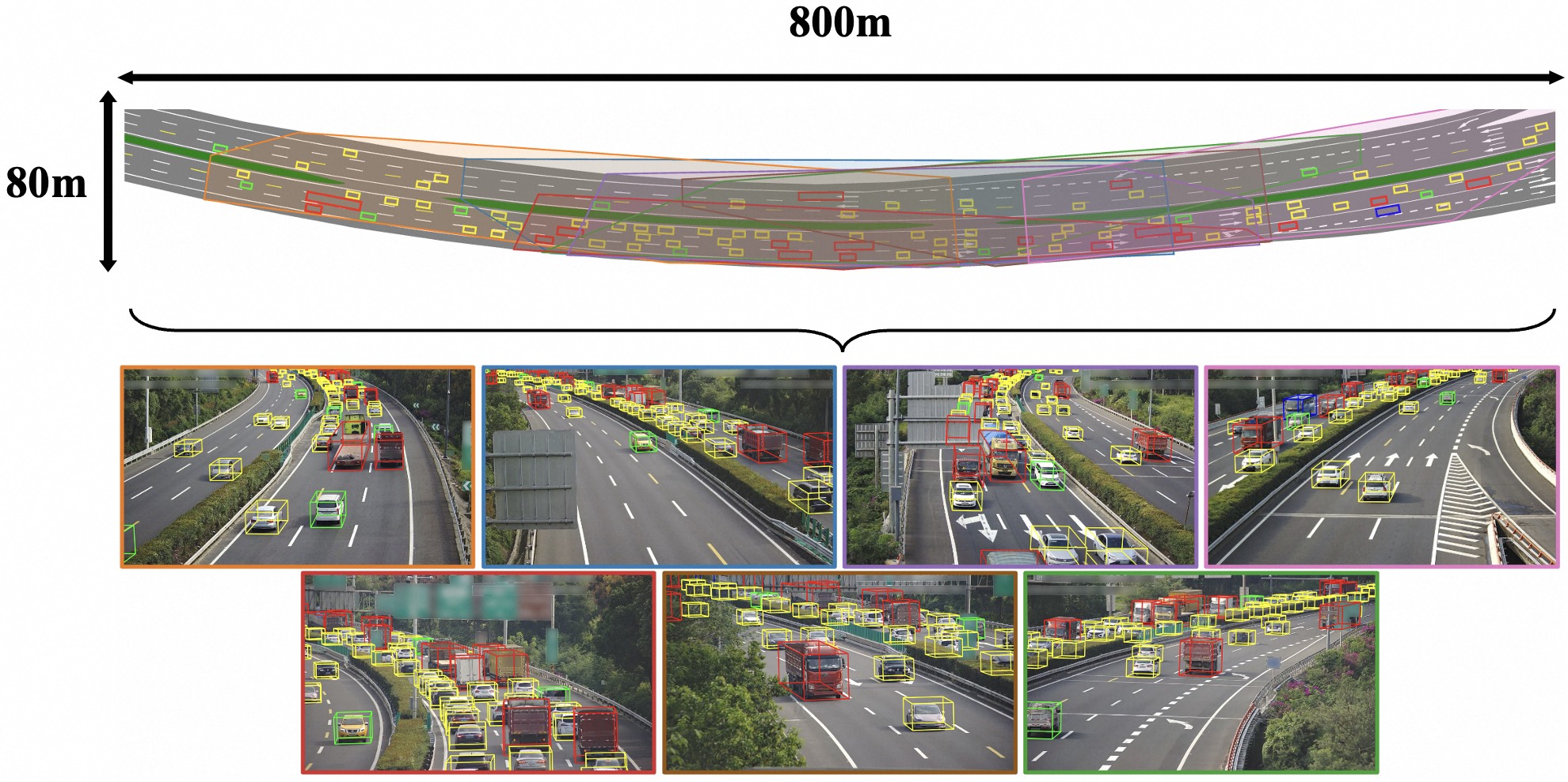
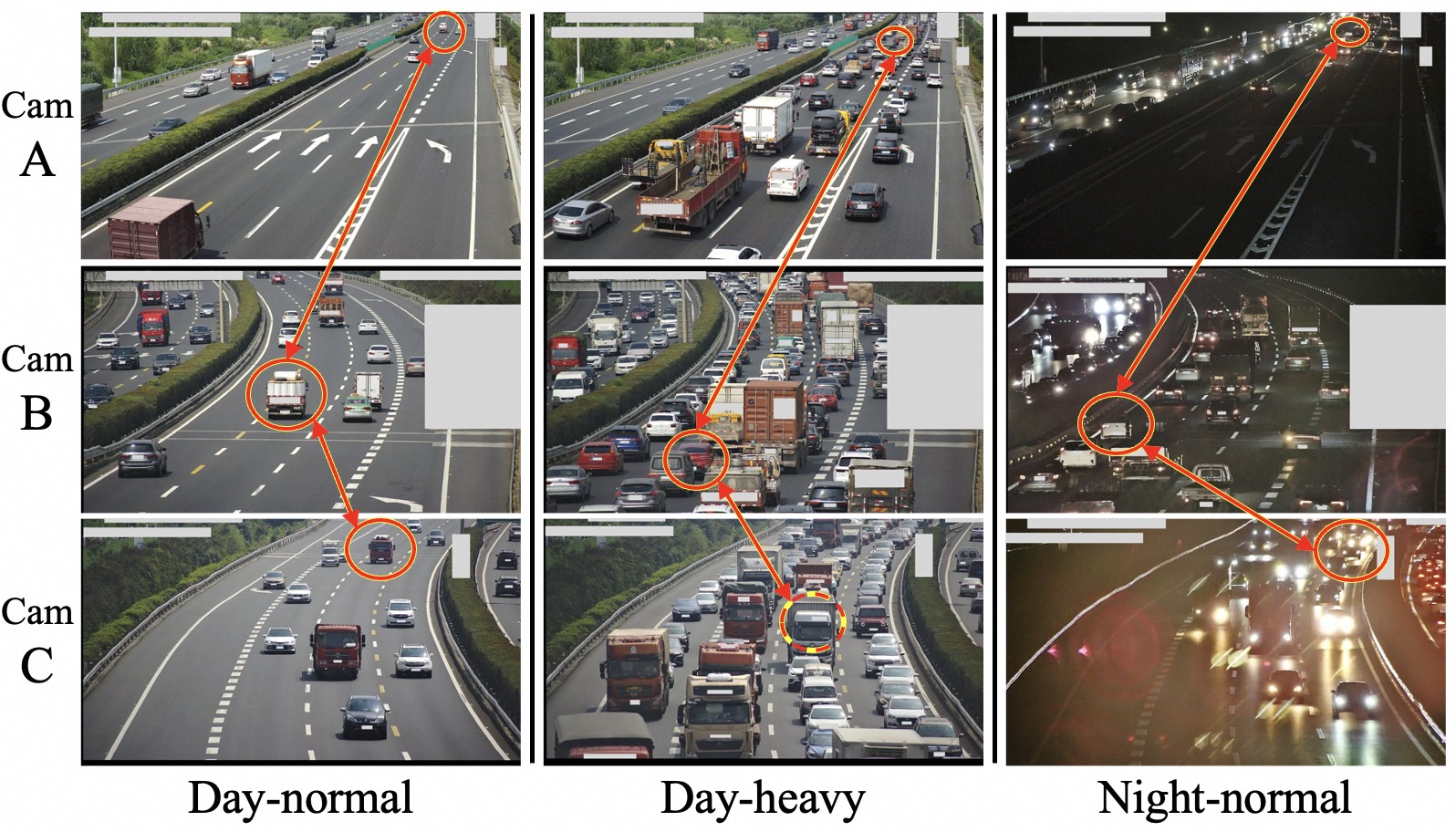

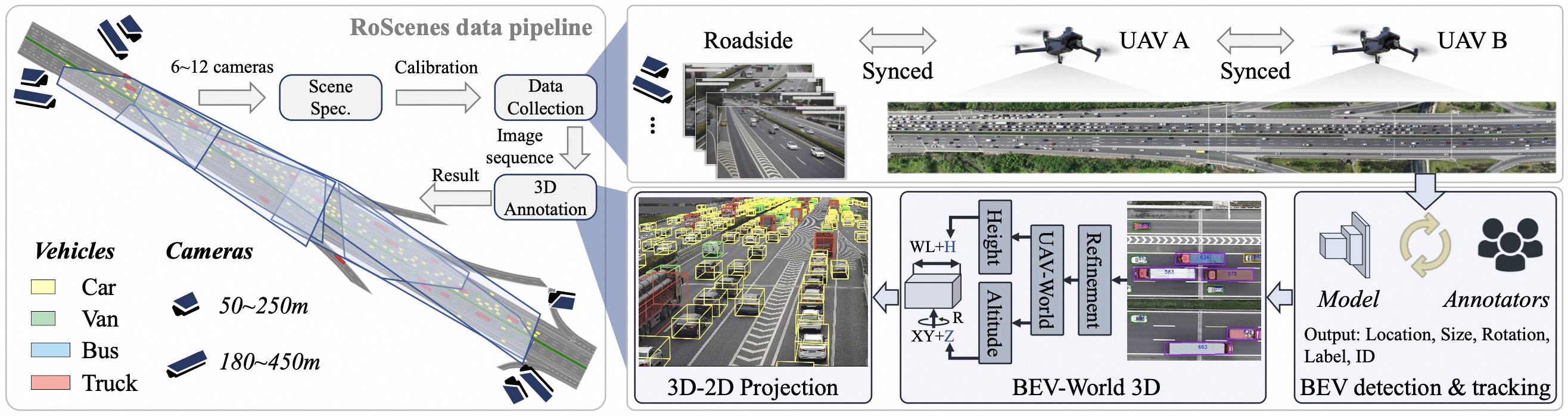
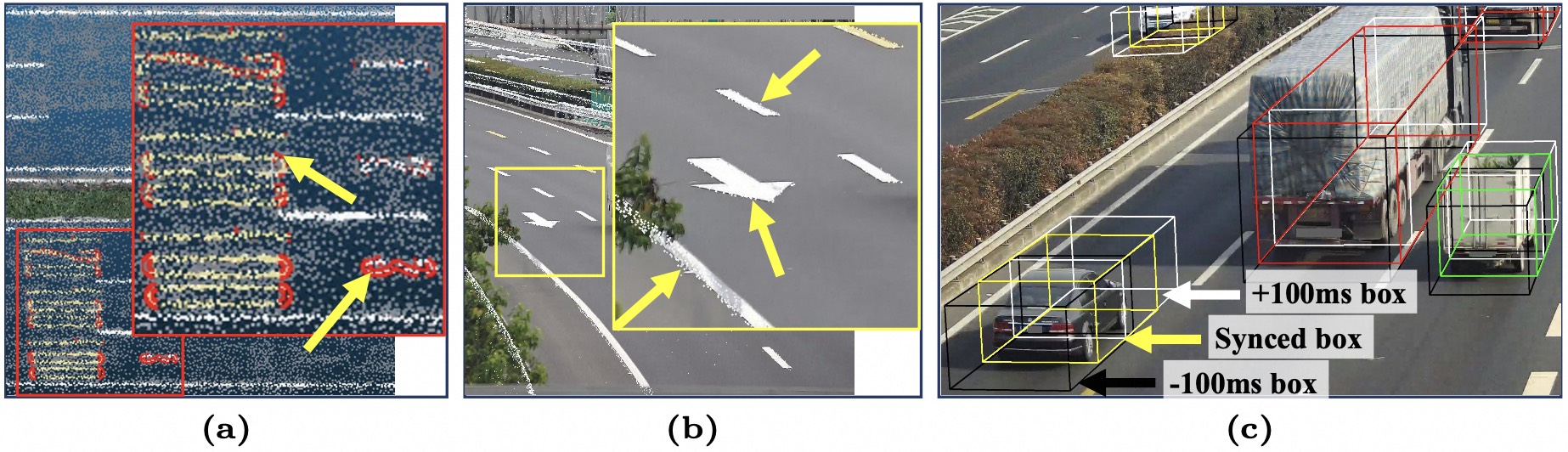
(b): Calibration and projection error visualization. We select a camera and pick a single frame as background, and project white points sampled from 3D reconstruction to this perspective view as overlay.
(c) Vehicles' location and height error. To avoid temporal disalignment and height mismatch, we manually check the fitness of projected boxes with adjacent frames.

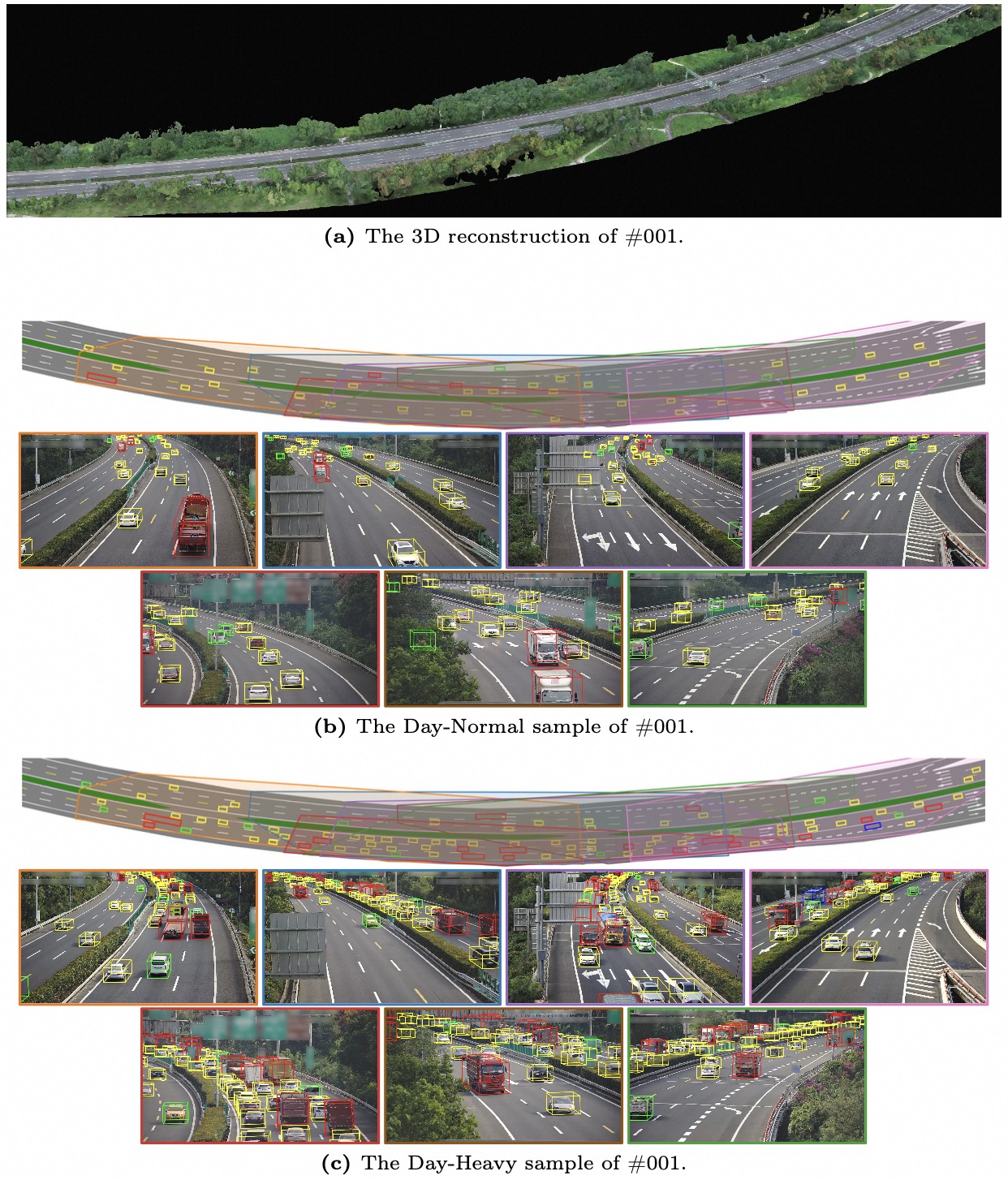
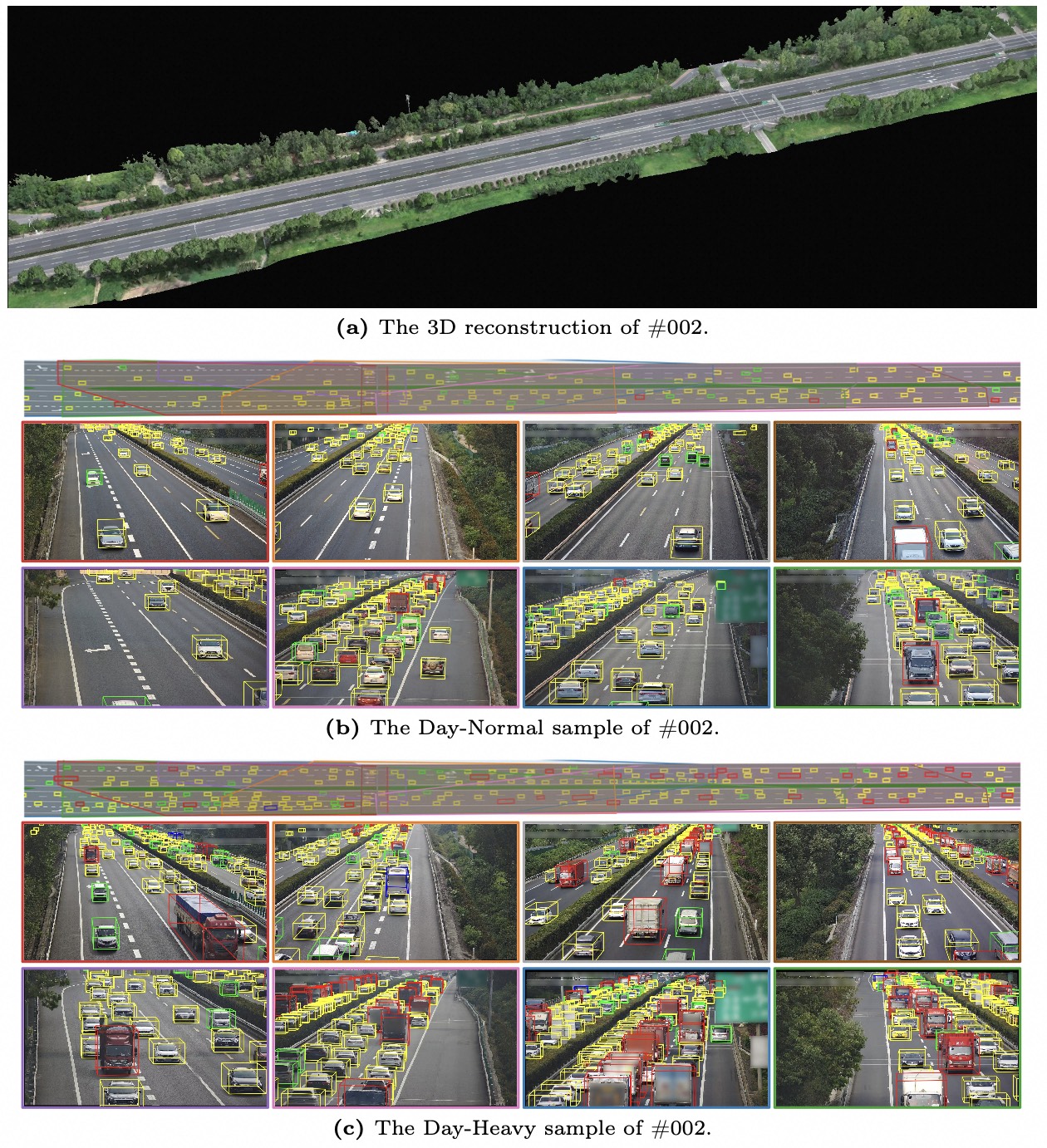
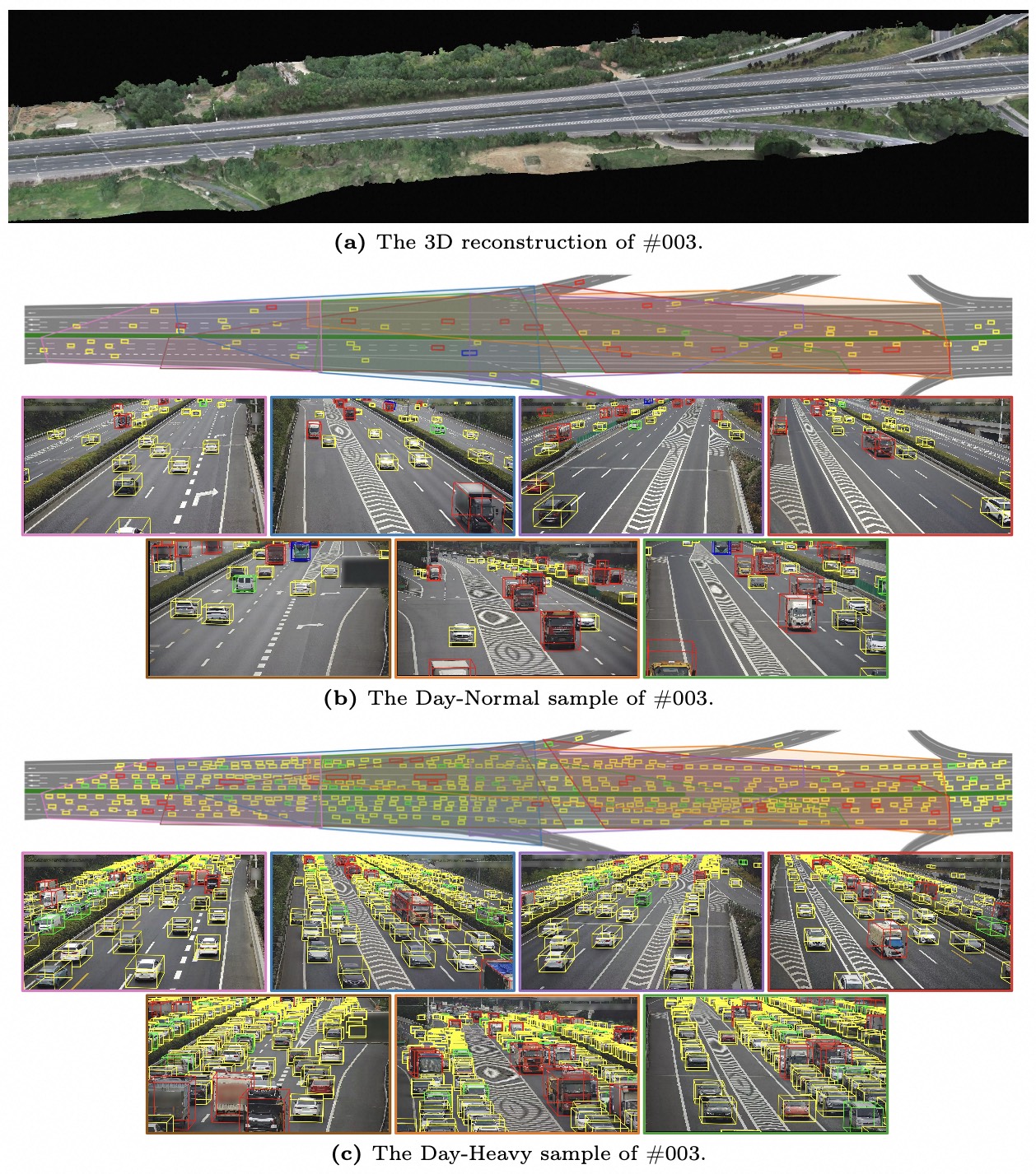
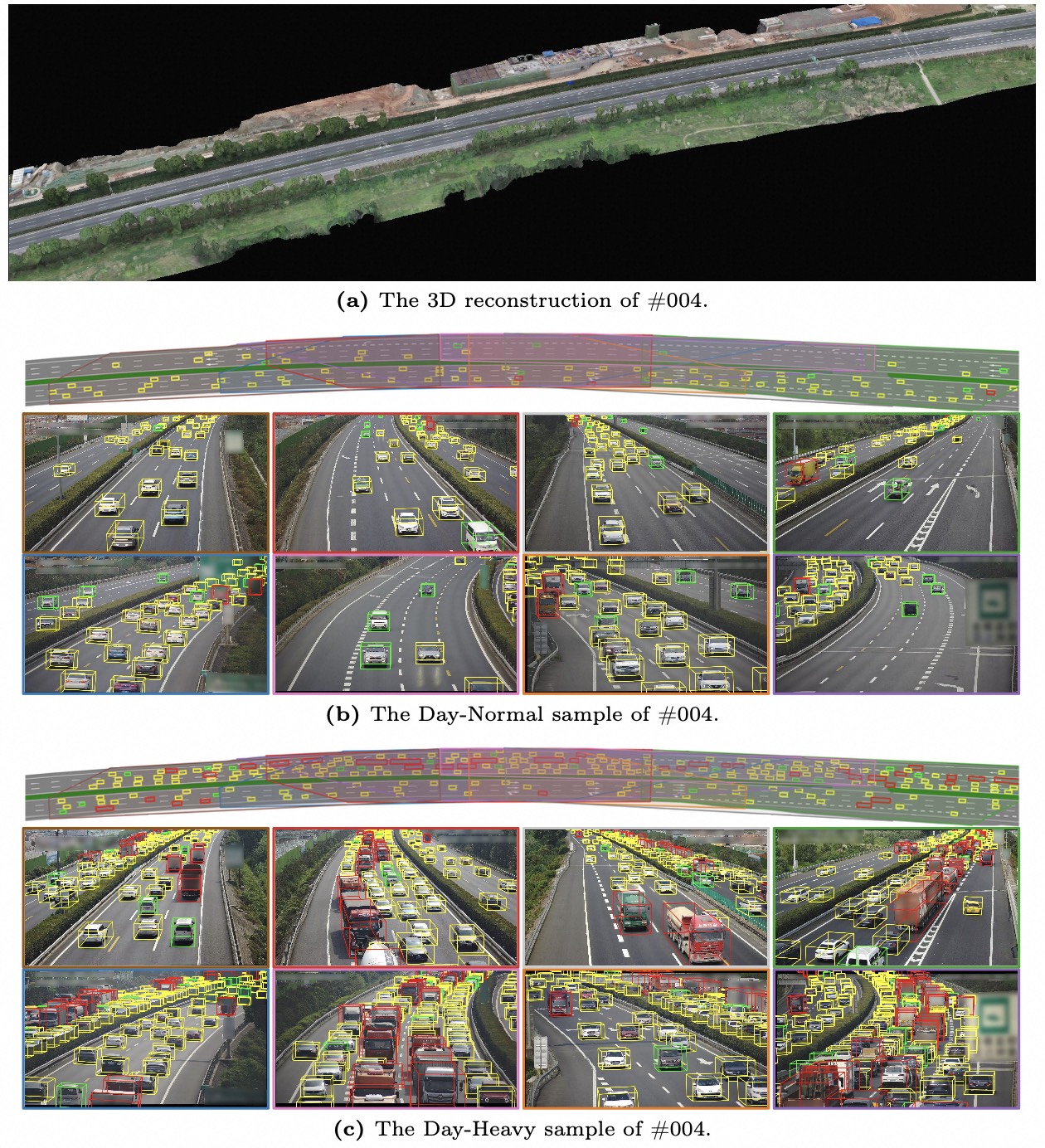
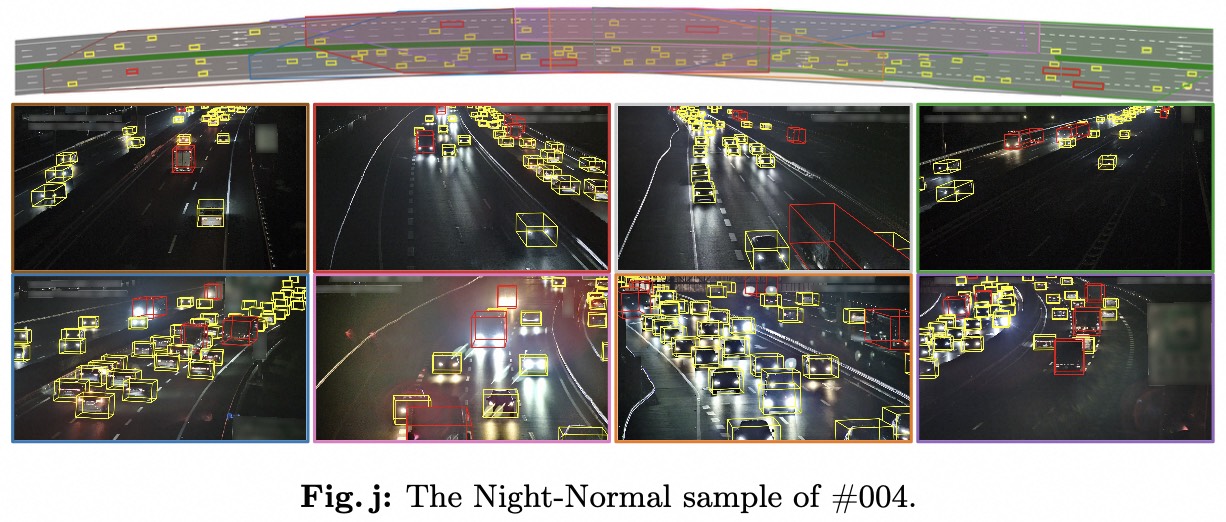